Abstract
Purpose
To investigate the performance of deep convolutional neural networks (DCNNs) for glaucoma discrimination using color fundus images
Study design
A retrospective study
Patients and methods
To investigate the discriminative ability of 3 DCNNs, we used a total of 3312 images consisting of 369 images from glaucoma-confirmed eyes, 256 images from glaucoma-suspected eyes diagnosed by a glaucoma expert, and 2687 images judged to be nonglaucomatous eyes by a glaucoma expert. We also investigated the effects of image size on the discriminative ability and heatmap analysis to determine which parts of the image contribute to the discrimination. Additionally, we used 465 poor-quality images to investigate the effect of poor image quality on the discriminative ability.
Results
Three DCNNs showed areas under the curve (AUCs) of 0.9 or more. The AUC of the DCNN using glaucoma-confirmed eyes against nonglaucomatous eyes was higher than that using glaucoma-suspected eyes against nonglaucomatous eyes by approximately 0.1. The image size did not affect the discriminative ability. Heatmap analysis showed that the optic disc area was the most important area for the discrimination of glaucoma. The image quality affected the discriminative ability, and the inclusion of poor-quality images in the analysis reduced the AUC by 0.1 to 0.2.
Conclusions
DCNNs may be a useful tool for detecting glaucoma or glaucoma-suspected eyes by use of fundus color images. Proper preprocessing and collection of qualified images are essential to improving the discriminative ability.




Similar content being viewed by others
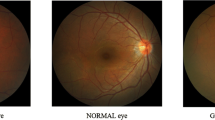
References
Quigley HA, Broman AT. The number of people with glaucoma worldwide in 2010 and 2020. Br J Ophthalmol. 2006;90:262–7.
Iwase A, Suzuki Y, Araie M, Yamamoto T, Abe H, Shirato S, et al. The prevalence of primary open-angle glaucoma in Japanese: the Tajimi Study. Ophthalmology. 2004;111:1641–8.
Gulshan V, Peng L, Coram M, Stumpe MC, Wu D, Narayanaswamy A, et al. Development and validation of a deep learning algorithm for detection of diabetic retinopathy in retinal fundus photographs. JAMA. 2016;316:2402–10.
Ting DSW, Cheung CY, Lim G, Tan GSW, Quang ND, Gan A, et al. Development and validation of a deep learning system for diabetic retinopathy and related eye diseases using retinal images from multiethnic populations with diabetes. JAMA. 2017;318:2211–23.
Cerentini A, Welfer D, Cordeiro d’Ornellas M, Pereira Haygert CJ, Dotto GN. Automatic identification of glaucoma using deep learning methods. Stud Health Technol Inform. 2017;245:318–21.
Li Z, He Y, Keel S, Meng W, Chang RT, He M. Efficacy of a deep learning system for detecting glaucomatous optic neuropathy based on color fundus photographs. Ophthalmology. 2018;125:1199–206.
Anderson DR, Patella VM. Automated static perimetry. 2nd ed. St. Louis, MO: Mosby; 1999.
Simonyan K, Zisserman A. Very deep convolutional networks for large-scale image recognition. Paper presented at: International Conference on Learning Representations; May 7–9, 2015; San Diego, CA.
He K, Zhang X, Ren S, Sun J. Deep residual learning for image recognition. In: Proceedings from the IEEE Conference on Computer Vision and Pattern Recognition; June 27–30, 2016; Las Vegas, NV.
Huang G, Liu Z, van der Maaten L, Weinberger K. Densely connected convolutional networks. In: Proceedings from the IEEE Conference on Computer Vision and Pattern Recognition; July 21–26, 2017; Honolulu, HI.
Zhou B, Khosla A, Lapedriza A, Oliva A, Torralba A. Learning deep features for discriminative localization. In: Proceedings from the IEEE Conference on Computer Vision and Pattern Recognition; June 27–30, 2016; Las Vegas, NV.
Sevastopolsky A. Optic disc and cup segmentation methods for glaucoma detection with modification of U-Net convolutional neural network. Pattern Recogn Image Anal. 2017;27:618–24.
Keel S, Lee PY, Scheetz J, Li Z, Kotowicz MA, MacIsaac RJ, et al. Feasibility and patient acceptability of a novel artificial intelligence-based screening model for diabetic retinopathy at endocrinology outpatient services: a pilot study. Sci Rep. 2018;8:4330.
Devalla SK, Chin KS, Mari JM, Tun TA, Strouthidis NG, Aung T, et al. A deep learning approach to digitally stain optical coherence tomography images of the optic nerve head. Invest Ophthalmol Vis Sci. 2018;59:63–74.
Murata H, Zangwill LM, Fujino Y, Matsuura M, Miki A, Hirasawa K, et al. Validating variational Bayes linear regression method with multi-central datasets. Invest Ophthalmol Vis Sci. 2018;59:1897–904.
Yousefi S, Kiwaki T, Zheng Y, Sugiura H, Asaoka R, Murata H, et al. Detection of longitudinal visual field progression in glaucoma using machine learning. Am J Ophthalmol. 2018;193:71–9.
Acknowledgements
This work was supported by a Grant for ICT infrastructure establishment and implementation of artificial intelligence for clinical and medical research from the Japan Agency of Medical Research and Development AMED. The study was performed as one of the works of the JOI registry group. A list of this group’s members is available as an Electronic supplementary material.
The members of The Japan Ocular Imaging Registry Research Group—Chairman: Tetsuro Oshika (Tsukuba University); Steering Committee Members: Takashi Hasegawa (Japanese Telemedicine and Telecare Association), Kenji Kashiwagi (Yamanashi University), Masahiro Miyake (Kyoto University), Taiji Sakamoto (Kagoshima University); Members: Takeshi Yoshitomi (Akita University), Masaru Inatani (Fukui University), Tetsuya Yamamoto (Gifu University), Kazuhisa Sugiyama (Kanazawa University), Makoto Nakamura (Kobe University), Akitaka Tsujikawa (Kyoto University), Chie Sotozono (Kyoto Prefectural University), Koh-Hei Sonoda(Kyushu University), Hiroko Terasaki (Nagoya University), Yuichiro Ogura (Nagoya Prefectural University), Takeo Fukuchi (Niigata University), Fumio Shiraga (Okayama University), Kohji Nishida(Osaka University), Toru Nakazawa (Tohoku University), Makoto Aihara (Tokyo University), Hidetoshi Yamashita (Yamagata University), Iijima Hiyoyuki (Yamanashi University)
Conflicts of interest
S. Phan, None; S. Satoh, None; Y. Yoda, None; K. Kashiwagi, None; T. Oshika, Grants (HOYA, Abbott Medical Optics, Alcon, Kai, Novartis, Pfizer, Santen, Senju, Topcon), Speaker Honoraria (HOYA, Abbott Medical Optics, Alcon, Santen, Senju, Tomey, Otsuka, Kowa, Japan Focus), Consultant fees (Alcon, Mitsubishi Tanabe, Santen), Equipment Supply (Tomey).
Author information
Authors and Affiliations
Consortia
Corresponding author
Additional information
Publisher's Note
Springer Nature remains neutral with regard to jurisdictional claims in published maps and institutional affiliations.
Corresponding Author: Kenji Kashiwagi
Electronic supplementary material
Below is the link to the electronic supplementary material.
10384_2019_659_MOESM1_ESM.pdf
Supplemental Figure Representative images defined as poor-quality images are shown. a YMU-gla, b KOSEI-gla, c KOSEI-normal (PDF 121 kb)
About this article
Cite this article
Phan, S., Satoh, S., Yoda, Y. et al. Evaluation of deep convolutional neural networks for glaucoma detection. Jpn J Ophthalmol 63, 276–283 (2019). https://doi.org/10.1007/s10384-019-00659-6
Received:
Accepted:
Published:
Issue Date:
DOI: https://doi.org/10.1007/s10384-019-00659-6